Navigating the AI Landscape in Financial Institutions with Shekhar Vemuri
“Banks have been going through this digital journey in some shape or form. First to automate the core system, the back office, ledgers, and making sure that everything reconciles. Then it started going to building muscles so that the rest of the body, which is all the operations, the function of productivity gains, bringing in efficiency in processes. And then the last phase being improving customer experience.”
Episode Summary
In this episode of the Digital Banking Podcast, Josh DeTar spoke with Shekhar Vemuri, SVP, CTO, VP Analytics at EXL Analytics. Vemuri shared insights on the evolution of AI in banking and its practical applications.
Vemuri explained how AI has progressed from basic automation to more complex tasks like understanding unstructured data. He highlighted the shift from optical character recognition (OCR) to advanced machine learning models that can comprehend and analyze documents.
The conversation delved into the challenges and opportunities of implementing AI in banking. Vemuri stressed the importance of balancing innovation with risk management, especially in highly regulated industries. He also discussed the role of human oversight in AI-driven processes, emphasizing that while AI enhances efficiency, it doesn’t replace human decision-making entirely. The episode provided a comprehensive look at how AI is reshaping banking operations and customer service.
Key Insights
⚡ AI’s Evolution: From Simple Automation to Creative Problem-Solving
AI has advanced from basic automation to complex problem-solving. This shift allows AI to understand context, interpret unstructured data, and generate creative solutions. In banking, AI now comprehends customer queries, analyzes loan applications, and assists in decision-making processes. The technology’s ability to process vast amounts of data and learn from it has transformed operations, improving efficiency and customer experience. However, this evolution also brings challenges, such as the need for careful implementation and human oversight. As AI continues to develop, it will likely reshape many aspects of financial services, from customer interactions to risk assessment and product development.
⚡ The Human Element Remains Crucial in AI Implementation
Despite AI’s rapid advancement, human involvement remains essential. AI systems, while powerful, are not infallible and can produce errors or “hallucinations”. In high-stakes industries like banking, human oversight is crucial to ensure accuracy and manage risk. Professionals need to adapt their skills to work alongside AI, focusing on tasks that require judgment, empathy, and complex decision-making. The future of work in banking will likely involve a symbiotic relationship between AI and human expertise, with AI handling routine tasks and data processing while humans provide strategic direction and ethical considerations.
⚡ Balancing Innovation and Risk in AI Adoption
Implementing AI in banking requires a delicate balance between innovation and risk management. While AI offers significant potential for improving efficiency and customer service, it also introduces new risks, such as data privacy concerns and potential biases in decision-making algorithms. Financial institutions must carefully assess where and how to apply AI, considering regulatory compliance and customer trust. This balance involves experimenting with AI in lower-risk areas before expanding to more critical operations. As AI technology evolves, banks will need to continually reassess their strategies, ensuring they leverage AI’s benefits while maintaining robust risk management practices.
About The Guest
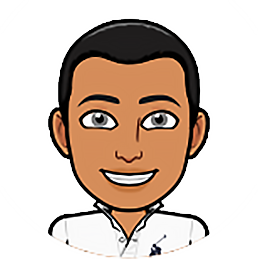
Adrenaline junkie and space enthusiast driving AI innovation in banking.
Shekhar Vemuri: [00:00:00] it’s taking a step back and saying, okay, it’s AI in its whole, and there’s different, you know, it’s never going to be only Gen AI or only machine learning of certain kind, predictive analytics, whatever we want to call it, right?
Or whatever terms we’ve used in the past, it’s really, how do you bring all of this together? And, in certain instances, it’s going to be, generative AI. In certain instances, it’s not, you could just go build something really well, just based off of some rules and heuristics that you know.
[00:01:00] You know, your podcast hasn’t officially made it until there’s ads in it. But this is one you’re not going to want to skip past. And, well, if you do, feel free to hit that fast forward 15 seconds button twice. Ever wonder what gives me my energy and enthusiasm during these podcasts? You know, outside of my relentless desire to learn about, connect, share, and build up community FIs and their mission to support the communities they serve?
It’s coffee. And lots of it. Now, you want to know what’s better than your regular old coffee? How about donating 5 to the Children’s Miracle Network Hospitals through Credit Unions for Kids every time you purchase high quality, [00:02:00] ethically sourced coffee that also provides living wages to coffee farmers.
So if you want to listen to this episode with epic levels of caffeine induced focus and help kids in need, head to java4kids.org to learn more and buy a bag or ten. Thanks.
Josh DeTar: Welcome to another episode of the digital banking podcast. My guest today is Shekhar, the SVP CTO of analytics for EXL analytics. Now a direct quote from Shekhar, adversity is an opportunity. Now that’s a pretty loaded statement, but I think it has a lot of merit to it. We grow by being challenged.
For Shekhar, putting himself in uncomfortable situations is a tool to force growth, change and adaptation. What’s that old saying? What doesn’t kill you only makes you stronger, right? Well, apparently that works well for Shekhar. he said he’s a total adrenaline junkie and even jumping out of a plane was for him pretty [00:03:00] anticlimactic actually.
Being up high gave him beautiful perspective and didn’t really get exhilarating until the ground started to get a little closer. Hence, why the next thing he wants to try is bungee jumping. This new adventure seeking side of Shekhar’s personality extends deep into his professional life as well. He refuses to be put in a box, wants to explore, try new things, and take risks.
Having spanned the tech ecosystem on many fronts, as well as being on the entrepreneurial side of intersecting business, tech, and finance, Shekhar has a wide lens to view things from. As someone who is fascinated by space, and even at one point as a child had aspirations of being an astronaut, Shekhar is looking into the unknown, trying to solve problems, looking for opportunities in front of us with the rate and velocity of technology change, especially around AI and the movement from computers being [00:04:00] Pretty good at doing what we asked them to do to actually knocking on the door of creativity and them actually being able to think for themselves a lot like space. There’s a lot we know and a lot we don’t. Whether it’s in the front of the line for a roller coaster.
cooking, listening to the latest from Neil deGrasse Tyson, or taking a much needed pendulum swing to the other extreme and lazing on a beach and recharging. Shekhar is keeping things interesting. And I’m going to be honest, we can expect no less from him in today’s episode. So Shekhar, thanks so much for joining me as a guest on the podcast.
Shekhar: Josh, pleasure to be here. Thank you for having me,
Josh: I got, I mean, it was kind of funny when I was talking to you and, I got to admit, right? Like you talk to a really well presented professional tech guy who’s got, you know, the resume to back this all up and I ask you like, what’s, you know, what’s your personality like? And you’re like, Oh, I’m an adrenaline junkie.
Like I’ve lobbed myself out of an airplane. [00:05:00] I’m like, wait, what? Hold the phone. And I thought was even crazier was when you’re like, Oh yeah, no, actually, you know, skydiving was pretty anticlimactic. I think that is kind of a good indicator of like you really are kind of trying to look for, what’s, what’s on the edge of things, right?
Whether that’s just in kind of the personal sense or the professional sense. but where did this like curiosity for adrenaline junkie start?
Shekhar: Well, it wasn’t from day one for sure, so I think, uh, it really intersected with, you know, as sometimes. It takes a little while to kinda find your way, from the perspective of like, or you, you do what you do and then, one kind one day it kinda clicks when you see, a direct link between what you do and the impact that it creates.
And I think, uh, I know it’s been a while, but 15, 16 plus years ago, I think that was the thing that kind of clicked in my head in terms of [00:06:00] like, Oh, I’m making difference. I’m making an impact. It’s not just, the mechanics of doing something. it’s really. Uh, especially with software, you can kind of, you know, in the technology field, solve problems, in fairly interesting ways and, take different techniques and bring them together.
So I think, uh, a few years ago, that’s what happened. And ever since it was like, Oh, I just can’t have enough.
Josh: That’s cool that you kind of found that correlation though between, kind of that personal adrenaline junkie side and the professional and even just the, like, being a human on this planet kind of side of things of, okay, where are the areas where I can kind of push the boundaries and, and have some fun.
But it’s also whether it’s making a positive impact on my life or someone else’s, like It’s impressive that you were able to kind of draw that correlation. so I want to talk to you about, the buzzword, right? Like [00:07:00] we, we drop it a little bit in, in the intro. and I’m going to be straight up like, this is the reason why I wanted to have you as a guest on the podcast because everybody’s kind of talking about it, but I’m always really interested to see different people’s perspectives because I think when we throw out that AI term, There’s a lot of marketing hype, and there’s a lot of people that are talking about it, but it’s the practical applications that are where the rubber really meets the road.
And so I really enjoyed having the opportunity to have some guests with different perspectives on that. And I really have appreciated kind of some of your perspectives. So let’s maybe just like level set all the way back to. You know, what does generative A. I. mean to you? What’s kind of the lens that you are applying to it?
And then let’s talk a little bit about, how you see some of the use cases for it. And, you know, you again, you made kind [00:08:00] of a comment to me that we put in the introduction for you. That’s, computers used to be really good at just doing what we told them. Now they’re starting to almost think for themselves.
Right? So what’s that going to look like? so yeah, if you could maybe just outline for me a little bit of like, what does that all mean to you? what’s your definition of the buzzword, I guess is my ask.
Shekhar: Great question. So, Where do we start? So, sofirst and foremost, just to get something out of the way. I mean, I always look at as much as there’s all this buzz about Gen AI, truly, I think it’s taking a step back and saying, okay, it’s AI in its whole, and there’s different, you know, it’s never going to be only Gen AI or only machine learning of certain kind, predictive analytics, whatever we want to call it, right?
Or whatever terms we’ve used in the past, it’s really, how do you bring all of this together? And, in certain instances, it’s going to be, generative AI. In certain instances, it’s not, you could just go build something [00:09:00] really well, just based off of some rules and heuristics that you know.
so just having said that, because, and part of the reason why I’m kind of clad, setting up that stage is because. To your point, buzzwords, easy to get carried away, right? even Gen AI, it’s been in the works for a while. Uh, it’s not something that, right? I mean, while of course, the democratization of it, the ease of access, uh, or, you got to give credit to open AI and ChatGPT to just open it up to everybody.
but it’s been in the works for a while and absolutely none, uh, maybe just a tad bit, uh, but it’s, for anybody who’s been in the space of, language, NLP, language comprehension, the, the whole space of, semantic anything semantic, right? Semantic search. Even a lot of these techniques have been around for a [00:10:00] while.
but a few things have kind of all, come together to create that watershed moment, right? So, first and foremost. just like anything else, great ideas, sometimes linger around till somebody has the right tools to put, you know, bring it to life. So, the way I see that is, it’s definitely compute, right?
uh, Because this, and we’ll talk more about this through the, through this conversation, but, it’s definitely compute hungry, right? So having that right compute, uh, having that right amount of data and then being able to bring the techniques, the algorithms. and, the data and now this compute to build something, that previously was probably conceived, but never really, you know, seen through that’s the paradigm shift that’s probably happened over the last, I would say.
Eight to nine years, right? So it’s been, while the foundations have [00:11:00] been around for a while, eight to nine years, are what I would, put the real revolution, attributed to that. And I go back to an, I missed one part. the other thing is also, this, thought process of, uh, applying engineering, right?
Everything is an engineering, thought process. so, so why do I say this? So Google, as you know, has at the forefront of AI for a long time. Google Brain did tons of R& D. fact, I think OpenAI the rest of the industry would be anywhere close to where it is. without all the stuff that, you know, Google research, but also more importantly, contributed back to the open source community.
But even Google talks about, how, as, you know, translate. google. com, right? We all use it to translate between languages. for the longest time. it used to be like, you know, a bunch of benchmarks where language translation is measured in comparison to a human and for the [00:12:00] longest time, it would come close, but not really, consistently, uh, surpass a human’s ability.
Right? And about nine years ago to somewhere in that 2015 2016 timeframe, it started flip to start it and started continuously surpassing human performance in those benchmarks. And that’s when even Google said, so what changed, right? While, of course, there was advancement in the way things were done advancement in the algorithms, the general research.
But one of the key foundational aspect in addition to the data and the, you know, having the right hardware compute was having this engineering mindset, right? So, moving from the, just thinking about the math of it all to just saying, Hey, I’m gonna go, engineer this, try out a bunch of iterations, see what works best, learn from it and just keep on iterating over it crazily.
So, [00:13:00] and that’s what kind of unleashed, the improvement. so coming back to your question, you know, while there’s enough provenance over the last eight, nine years, all of it, but you know, everybody’s question is, okay, how does this impact. Two things, in what I do in my day-to-Day life, and of course professionally, right?
So, Generative AI, the way I see it, slightly different from a bunch of the other, stuff that we’ve seen materialize in or come up in the past. think we are really, uh, you alluded to this in the, intro, we’re going from where we just tell computers what to do, and that’s all they do to, what seems to be like at the, you know, At the beginnings, very, very early days, maybe Big Bang plus, you know, uh, one millionth of a second, kind of timeframe, of how we are unlocking creativity, right?
Of course, [00:14:00] now, all of this is still learned from every piece of content, every piece of art, every piece, every image, every video that we as humans have created, but I think, the unleashing that slightly creative and generative aspect, which was always a challenge in the past is truly where the paradigm shifts happening.
Right? And,
but that’s not just generative in nature, right? one of the other things that’s also happened is, comprehension, understanding, understanding conversations, and this is how, uh, this is where we’ll probably even take an example, something that we all, associate with, both as a consumer of this and sometimes, you know, as a builder is, let’s just take the example of, as much as people don’t call in, to get any kind of support, as much as they used to before.
But, you still, you know, call in occasionally. I, try to do as much as I can, via. A, you know, [00:15:00] text message or via apps or whatever, when you need customer service agent, you need 1 and when you call them, the, the ability to understand that conversation to ability to comprehend. What’s going on?
And this is I’m not just talking about base sentiment analysis. Was Shekhar upset on the call? Or was he, happy? Was he, you know, did he sound satisfied? All that? That’s that’s great input. But really understanding. Okay, what? What’s the crux of the problem here? Right? What is the challenge that whoever’s calling in is running into?
and then how do you then. kind of marry it or stitch it to the solutions, that that you already know of, right? As, as an enterprise uh, as a company provider, delivering the product, long. has been the bane of customer service. The fact that I ask a question, somebody’s gonna be like, oh, let me just, you know, [00:16:00] go search for what your problem is and it will then, uh, something will pop up.
I’ll kinda ask you, oh, is this what you’re running into? Why did you try this? Try that, right? But, a simple but very powerful, capability of comprehending what I just said. And then being able to marry it to the, you know, the solutions that are already there, in your knowledge base. And then suggesting this to back to the, person who’s on the, uh, hand taking my call to the agent.
It’s, it’s, that is the paradigm shift, right? it’s, you’re not just doing basic search of what I just said. You’re actually understanding. So I could be expressing my, problem in 10 different ways. but still, the way you, uh, articulated the way I articulated. Somebody may have a, you know, incomplete information.
Somebody may have accent, all of it, but still leading towards the same solution. That’s [00:17:00] the power, right? Everybody’s seen the generative aspects of this. Hey, I’m now able to create, images. I don’t know. Maybe, Josh question to you. Do you use. image generation tools for some of your blogs, maybe you do a bunch of content creators are doing that, right?
So, things that used to be extremely difficult on now, almost like 5 seconds of a prompt, whether it’s in ChatGPT or, anywhere else stability, it’s just. At your fingertips. So it’s really a big, big change. Something that we’ve not seen over the last 25 years.
Josh: Um, wow. get a little bit more from you on this topic because this is something I find really interesting. you know, you were talking about, we’re in the like, you know, one millisecond of after the big bang, in terms of just how much we’ve accomplished with AI versus I’m assuming what you’re extrapolating to [00:18:00] be, you know, the potential, Future and state of it, right?
But even before that, like there’s been stuff happening that’s what I’d love to learn a little bit more about. I mean, you know, I, I guess I’m fortunate enough to work around some people significantly more intelligent and more versed in this stuff than I am. And so, you know, I’ve heard them talking about what’s been happening in, A.
- and M. L. and large language models over the period of time. But even for me, right, like you kind of called it out like ChatGPT was probably the first true democratization of some of those tools because I would say that there are absolutely people within my set, you know, circle. Um, That have been building and using those tools before ChatGPT.
I can tell you I was not one of them at all. And now I use ChatGPT multiple times a day, every single day. Right? So that was, [00:19:00] there was absolutely the catalyst for, that all of a sudden became a tool that I could, as an idiot, apply my use cases to and have it help me solve. And like you were saying, I mean, it would just, it kind of turned into like Google on steroids for me, right?
I had to think about, you know what? I may not know what I’m trying to accomplish, but I know how to ask the question. And so I can create a decent prompt in ChatGPT or other similar tools now, right? And it kind of helped walk me through it. So I was able to do that and say, Hey, I don’t quite know what I need, but maybe I, yeah, I need an image that’s something like this.
It looks kind of like this and maybe this style, or, Hey, maybe I need, a simple contract written up that makes sure that, these six areas are taken care of with, these specifications. Give me a good outline, right? And things like that, but like you were saying, I mean, this has been happening long before, so what [00:20:00] was happening before and why do you think now and why do you think it was ChatGPT?
Did this all of a sudden become so mainstream? Yeah,
Shekhar: Great. I actually happened to have a little bit of insight because, while the term generative AI is probably still more recent, at least the, the widespread usage of it, right? but a lot of this has roots in, NLP, natural language, comprehension, that whole space, right?
so over the years, let me take this example of a company. Yeah. So, this is from, six years ago, right? So, so there’s this company, uh, some of, listeners may have used it to, you know, that whole, uh, In a box trend. So especially after, what’s the dollar shave club and everybody came up with the subscription, models for, blades and, all of that.
but now, but that also went into the fashion industry. So there’s these companies [00:21:00] like Stitch Fix and a bunch of others. So every month or whatever frequency you want, you know, what they would do is, they would send, a box and you would just have like, a few pairs of clothes in there, right?
Shoes, accessories, a bunch of that. Now, why are we talking about this? because what I found interesting when I kind of, I just ran into, this, uh, person online, here, uh, used to write a great blog. I can’t remember the, the URL, but, uh, if I do, I’ll bring it up. But, x uh, I think nuclear physicist.
English major who then went into technology.
Josh: Yeah. That’s a totally natural progression. Can, can
Shekhar: many, psychology and physics and English majors I saw at grad school. So, there’s something about that, that, you know, none of us seem to be able to figure it out. but going back to the [00:22:00] example I was giving.
So, so he talked about how they use this, you know, so what they, how did they come up with this personalization, right? Because the game is two things. It’s, how do you in a digital context, almost, provide a personal shopper experience, So, the app or the website, all it took was like, you know, Hey, describe, describe your mood, describe your style, in some instances, maybe even, upload a couple of examples of, certain, clothes, uh, that you like or accessories, whatever, and describe them.
Right. A bit. So this was like, in those days, one of those early, representations of, Hey, you’re now starting to get into the, the realm where, text and images, and content in general was kind of You know, becoming fluid today. As you know, you can go from a prompt to an image and from an [00:23:00] image to a bunch of text in like a few seconds, right?
But back in the day, was still not that easy. So, but, coming back to that innovation that, that actually makes,What I do so exciting, it’s they, they used Bert and a few other, you know, libraries that Google and others techniques that, they had open sourced to really build this first, comprehension of text comprehension of content and even going back and forth between, text and images, right?
These these same folks used technology from a different space. which was used to go, index, huge amounts of content, right? So, vectorized con so vector databases is something, anybody who’s been in this, Gen AI space may have heard of. Uh, they were, they’ve been around for, uh,
Yeah,
Josh: explain that just a little bit for me?
Shekhar: yeah, absolutely.
so, but just before I do that vector [00:24:00] databases, some of the ones that have kind of shot through the roof and terms of usage, you know, they have provenance from like 10 years ago, right? They’ve been around for 9 to 10 years. A couple of them.think pinecones, Sometime 2010 2012, something about that. So now what’s happening behind the scenes right?
now there’s all this content on the Internet, going back to our Hey, what were the key ingredients that led to this, watershed moment, or, you know, kind of exponential growth? Of course. tons and tons of content, which, Hey, on the internet, whether it’s blogs, whether it’s, uh, reputed publishing, houses, some of whom may not be very happy with, open AI, as you can see in the news and the print, but, tons of content out there.
but how do you, you know, really, understand it in the past? He was like. Okay. if, if you just typed in Shekhar or something on Google or [00:25:00] Yahoo search, whatever it was, you would just match that against, you know, the web websites, the pages, all of it do, essentially token to token comparison, right?
the word to word and find results. Rank it in a certain way and send it back to you. but where the shift started happening is like, okay, that’s great. But then English specifically is so ambiguous, right? In, in the way it works, context is king. Without context, the same word, you don’t know what it, what it may mean or imply, are you talking about.
an apple pie, uh, or, uh, you know, geometry when you talk about pies, uh, what exactly are you talking about? So, so what changed is, you know, using techniques like vectorization, which all it’s doing is, you know, It’s even above my, head, but in a simple way, it’s just drawing out these, it’s representing text, on a [00:26:00] multidimensional, vector space, right?
So, so, but to simplify it, power of this, right, what happens is you can now do math. And the classic example here is, this is like the hello world of, for vectors is people figured out that when they applied these algorithms and techniques, they could now say, Hey, man, my, uh, plus, uh, yeah, king minus man equals queen minus woman, right?
So it’s almost like some, by virtue of, not just looking at keywords, by virtue of looking at all the content and knowing that the gender typically man, male, whatever is associated with king and, queen is associated with woman was a learning that came about right now. Why is this important?
Now I’ll go back to what I was saying. If you remember that [00:27:00] example that I was giving you, where I called into a call center, I had said. 10 different people could use different, ways of expressing the same thing, which probably has different meaning, but, at its core, ultimately is referring to the same thing, right?
So, that’s where, the proximity, when you map this out, on a vector space, let’s just take a 2D vector space, right? So if you, X and Y axis. what they found was in that space, man and king are very close to each other and woman and, queen are close to each other. Right? so similar concepts started clubbing together and hence you could then say that, okay, if you were referring to.
Pie and apple very different places. You’re probably talking about the recipe as opposed to talking about geometry or something else. So lots of again. I think as much as [00:28:00] November 23 or so was the watershed moment, but a lot of work. I mean, all of this. Standing on the giants, standing on the shoulders of all the work that, you know, including Google, sometimes it doesn’t get enough credit for this, a lot of these folks both did the research and also, most importantly, open sourced it for everybody else to build on top
Josh: that makes a lot of sense. I’m glad you kind of went down the path of talking about the whole, king man, queen woman. So, one of the things I’ve heard, our CEO Siva talk about is just how these dimensions are getting so much deeper in their understanding and being able to match those things.
And he uses, I think, a similar example of, like, if you say a cat is very similar to a dog, you’re like, well, I mean, I guess they’re both animals. What is that relative to? And he’s like, well, if you apply it, a [00:29:00] cat is more similar to a dog than a tree. All you would need is one dimension to say, how many legs do they have?
The tree has none. Both the cat and the dog have four, right? So by that one dimension alone, they are now closer together than a tree. And that’s just one dimension. And now the number of dimensions that these AI models are getting to are exponentially greater. And you kind of alluded to this earlier that the change of pace for that is also crazy, right?
I don’t remember what the exact numbers are. I know Siva knows them, but, something to like, you know, the very first implication of ChatGPT to the general public and how many dimensions it could, it could look at versus how many it is now. It’s like mind blowing. Right in the new ChatGPT-4 versus the very first, installment of it.
And so, and that’s just in the course of basically a year. Right.
Shekhar: [00:30:00] yeah, barely a year, year and a half.
Yep.
Josh: that’s wild. But, but again, I want to go back again to the point that you were making of, it’s kinda like the tip of the iceberg though. In the sense is like, that’s what we’re seeing, but there was so much underneath that was happening.
And, I also wanted to go back to, you were kind of talking about the example of the like, stitch, online,
Shekhar: fix.
Yeah.
Josh: fix. Yeah, yeah. The retailer. And so, I mean, some of this stuff has been, crudely used quite successfully, I would argue, even back then. Right. I mean, I’m probably one of those people that probably should have signed up for something like that.
Shekhar: How do you think, how do you think I know about it?
Josh: Like my poor wife, I either live in a Tyfone t shirt and like Nike basketball shorts, or I don’t know about that.
Shekhar: Yeah, half, half my wardrobe [00:31:00] is just full of swag. So it’s
Josh: Um, but so, so there’s been lots of examples and I think that brings us back to the point of, So this has been happening a lot longer than I think the general public really even talks about.
And to be fair, I think this has even been going on a lot longer than a lot of technology folks in specific industries. have really been talking about, and I absolutely think that banking is one of those, right? Banking is one of those, industries where, in my humble opinion, and just the interactions that I’ve had, and I, again, I grew myself in this same group too, right?
Where we think that, like, AI kind of came out of nowhere, right? And in all reality, it didn’t, right? Like we’ve been working on this for a very, very long time, but it hit our radar very aggressively recently when things like ChatGPT came out and everybody started freaking out going, is this thing going to take my job?
And on top of that, the, okay, so this thing is out [00:32:00] there. How do I need to be using it in my industry? And again, that brings us back to the point of the use cases. Right. And how do you start to identify what are the use cases that I can use this for? And you were alluding to some, some really simple examples, right?
Of even just being able to understand, okay, if Josh calls into, you know, Alaska airlines and is saying, You know, it’s a problem that my flight is delayed. What’s the underlying thing that he’s trying to communicate? Well, he’s trying to get from Chicago to Portland as quick as possible so he can help put his kids to bed, right?
How do we identify that? And then very quickly search a database of potential flight options that he would be able to qualify for. And what’s the quickest way that we can get him home? And so as you started to talk about some of the Mhm. Use case examples that also opens up the next can of worms that I’d love to get your perspective on, which is this is only as good as the data that’s in there, [00:33:00] right?
And then it’s, it’s only as good as the data that’s in there, but then it’s also getting lots of new good data as it’s going through its processes, too, right? So then how are you running analytics on that to take the next level up of intelligence and further that use case? So, you know, you were talking about, just one of the big catalysts for, being able to make something like ChatGPT more prevalent is, is it just being able to be fed with data, right?
Being able to be fed with all of this information. Again, I think that’s a whole nother podcast of how that was done and who’s getting sued over what. And, uh, but let’s just park that to the side, but it is, it’s, it’s, you’ve got to feed it with good data. Right. So if, you know, if I call Alaska airlines and I’m trying to get home as fast as possible and all they can do is look at a very simple set of, you know, Alaska flights that go directly from Chicago to Portland [00:34:00] versus, you know, being able to very quickly search every single flight, whatever I’m using probably a, this is probably a rudimentary example to you, but it makes sense to me.
It needs all that data in there. It needs all of that information, but then it’s skill set is to be able to go over that in, like you said, a multidimensional approach versus just a matching approach of like a Google search or like the old school days of maybe like a, you know, Travelocity or something.
It’s being able to really look at this multidimensional and then, you know, be able to look at more scenarios. The way a human would, but with more knowledge and faster processing power than a human would. Is that fair?
Shekhar: yeah, think, uh, it’s, it’s really one of those things that’s so difficult to pin down, partly because, hey, we’re still kind of putting it to, uh, Even, yeah, putting it to use, , and learning [00:35:00] ourselves. Right. But, but in effect, yes, that’s, that’s exactly, how it is. I mean, even going back to the example, the, that you were talking about, the key thing being data, right.
I think right before in the beginning, when we started talking, uh, I was touching upon how. Over the last 25, 20, 23, 25 years,banks, let’s just take banks, banks in particular, have been going through this digital journey, right, in some shape or form, what was, it was first to, hey, automate the, you know, the core system, the back office, hey, ledgers, and, making sure that, everything, uh,reconciles all of that, to then started going to, okay, well, that was the brain, but then how do I, build muscles so that the rest of the body, which is all the operations, the function of productivity gains.
bringing in efficiency in [00:36:00] processes, all of that happened. and then of course, the last phase being, okay, wait a second. Uh, we’re making all this digital, but we forgot the customer. we forgot the, uh, the customer or the member. And, now we need to make sure that we improve their experience, right?
So that that whole journey with evolution we’ve gone through, and on the surface, I think we’ve done a fairly good job, but there’s still a few things that, that are good to call out. So first and foremost, I think along the way, it became, a couple of things, right?
What didn’t necessarily happen or what didn’t necessarily happen as, pervasively as it could have is, while digital and digitization and automation happened, but it was in a lot of ways it was, Hey, what do I do today? How much of that is paper and how can I make it digital? Right? so and don’t get me wrong.
That was great, because at [00:37:00] least you were creating the data footprint that then the future, innovation can be, can take, advantage of. But you’re still kind of leaving a lot of, the benefits on the table, right? So, so what’s really happening now is going back to your question of use cases.
What is the application where does this become real? especially in a bank, in an enterprise, , small orbit for that matter, is the ability to reimagine workflows, reimagine processes. We imagine ways of doing work, right? So let’s just take this example. Classic one. So everything’s digital, but everything’s still some form driven or, you know, whether it’s paper or, online.
Right? now, ultimately, What has digital done? It’s taking something from one stage to from one human to another, to another, to another. And then once it [00:38:00] reaches enough context and knowledge, maybe goes to an AI model or a predictive model. and. Outcomes the output, right? But, let’s take that hopping from one human to another, right?
Passing along the work as an example. So, so what’s happening there and why was that the case? and how is Gen AI changing that? So loan applications, mortgage, I’ve worked for, a company that used to help a bunch of banks manage. just the documents, submitted during these, applications, application processes, and man, that’s all they did, right?
Just manage, it’s a document repository, couple of billion dollars of valuation, but the challenge all was who’s gonna, somebody still got to read it because a lot of them were, or scanned or sometimes, you know, poor quality, some handwritten stuff, [00:39:00] different formats, all of that.
Now, OCR came along, OCR helped quite a bit, but We all know the challenges of OCR, it’s great when it’s very specific, but stucks at being generic. so, so, uh, yeah, go ahead.
Josh: people listening, would you mind describing that a little bit more? So dive a little bit more into what OCR is. What does it
Shekhar: Yeah, sure.
Josh: with it? Because I think that’s a part of this equation.
Shekhar: No, absolutely. Yeah. So OCR, optical character recognition, right. it’s an offshoot of the computer vision space, but, essentially, humans are great. And this is this is the real paradigm shift, right? So I’m glad you even, pointed this out. But, what seems exceedingly simple to us sometimes.
uh, whether you know, you look at a form, you can read it, you can, understand it, grok it, you can, uh, you can see what’s the question, which is, hey, name, phone address, all of it, you can see, [00:40:00] decipher what the responses or the, stuff that was filled out was, but, put that in front of a computer that’s never seen that, uh, Before as, uh, dumb as it may sound sometimes that’s it’s extremely difficult for that machine to comprehend all of that.
Right? So, this is that whole last frontier of unstructured data. that’s out there, which there’s an insane amount of unstructured data out there, orders of magnitude, more than even structured data within a bank, right? just imagine all these forms, applications, everything that are just stored away in these repositories.
so
Josh: See, this is where, so now you’re talking like, so now you’re becoming the human that thinks it’s so easy for the rest of us because it’s easy for you. So when you talk about that, like, so what’s the difference between structured and unstructured?
Shekhar: absolutely. Yeah. Yeah. So, great [00:41:00] question. So structured data. it’s essentially. in the sense, so if you’ve anybody who’s worked with Excel, right, most people have played around with Excel,you’ve if you’ve ever done budgeting or something, you categorize your expenses, right?
So, so there’s usually, okay, you’ll create a table, it’ll have, okay, the date of expense. the type of expense, the category, and some description around it. Let’s just take a simple example, right? Now you would create a table where the headers would be there, right? So the date, the category and the description.
and then, rows would just be the values, right? The actual things that you fill up in the cells. Now, that’s a great example of structured data, because, you know, anytime you look at a cell, you can triangulate and say, Hey, what was this? Oh, it was the date of purchase, right? Just it’s very [00:42:00] descriptive.
It’s very, uh, specific in nature. and you won’t find, you know, the description of the purchase in the, date of purchase, unless, you know, something went horribly wrong somewhere. So, that’s very structured. That’s easy to, understand, not just for us, but most importantly for computers, right?
Because that’s where, They can now say, Hey, I can do math all around humans for all of this data because understand what it was. they can calculate the differences between, two dates. Super quick. What was the minimum purchase, uh, minimum purchase price, maximum purchase price, most expensive thing, average, all of this stuff becomes so easy.
But now suddenly. If I just typed up, uh, let’s just say, I did something like, Oh yeah, great examples. Now credit card statements. why don’t we use this, you know, same similar paradigm, credit card statements, [00:43:00] credit card, You know, one thing that I’m almost sure is universally hated is how concise those, descriptions becoming in the credit cards.
You know, sometimes you’re like racking your brains are like, wait, where did I spend this money? Right. What did this makes? Exactly. Yeah, they’ll, they’ll say, Oh yeah, Walmart sometimes becomes WMRT, WLMRT, all kinds of stuff happens, right? So, now if you take a credit card statement and you tell a computer, Hey, just like that budget Excel that we built, now go populate the budget Excel automatically.
Yeah. Okay, it knows what it needs to store it in. But when it looks at that credit card statement, all of that information is in there, right? There’s a purchase price, there’s a date, uh, there’s a description, there’s due dates of when you have to [00:44:00] make payments, all of it’s there.
But, it’s all, unstructured as in there’s no fixed format to it, right? Chase Bank’s credit card statement may be different from Bank of America. now even they may keep and it could be different from Amex and each of them may keep changing it. every few months to, you know, add a little bit information, take away some information, stuff like that.
So there’s no fixed format. There’s no fixed way of representing that. So that falls more in the unstructured, space, right? So. So the last frontier from a digital data perspective has been how do you handle unstructured data at scale? How do you, understand it? Actually, how do you, read it first?
Right? So let’s just say read it. Read being okay, this. this particular area is talking about the purchase price. This is talking about the description, [00:45:00] so on and so forth. Then once you read it, understand it and then use it just like any other structured data. it’s a problem and that I’ve been on both sides of solving and also experiencing for the last decade and a half.
And it’s only now, it’s only now that, I’m seeing solutions that are getting really good at solving that problem are becoming commonplace, right? I think in a couple of years, this will become a lot better, but whether it’s the like the cloud providers, AWS, Google, Microsoft Azure, Everybody has services that make, the reading and comprehension of unstructured data a lot easier than what it used to be even three years ago.
And lo behold, Gen AI has a key, you know, uh, part to play there because it’s also making a lot of this stuff easier to, understand.
Josh: And so how, [00:46:00] what’s the role of, you were talking about kind of the challenge of OCR and layering the challenge of OCR with the challenge of structured versus unstructured data. So how is that changing?
Shekhar: Yeah. So, going, we were talking about that loan process, right? So, now the challenge was, to automate it, that in the digital, you know, era, all it meant was I’ll scan it, I’ll store it in some place and whenever a loan officer or, uh, processor, whoever it is, underwriter in some instances.
comes in, they’ll get, you know, either a, a web page or something where they can then see the details of the application and all these documents that were submitted. Right? But what were they doing? They were still reading all of that, right? They couldn’t know. I mean, the technology wasn’t giving them.
Oh, by the way, so and so person has XYZ in assets. Uh, oh, by the way, we [00:47:00] looked at all of their credit card stuff and they have so much debt. ultimately biggest, input into, the ability to pay back loans is what’s your income, what’s your debt, right? so people had to go read through that, you know, extract all that information.
Somebody sometimes, even did manual data entry. So people would come in and, look at those things because it underwriters loan officers were very expensive. Right? So, Somebody who was maybe, you know, an intern or a bunch of other folks would come in and digitize and extract all the information.
So this is where the whole back office from a, BPO perspective and all of it went through the roof because somebody ultimately needed to look at this and, read it and then create the structured representation of all that. So, that’s the past though, right? Now what’s possible today?
What’s happening today? Today, two [00:48:00] things have happened now. OCR has been supplanted with, uh, A lot of machine learning models, which have become really good at detecting this because there’s so much written content out there, right? Ultimately, machines, they only learn from, you know, whatever’s out there and whatever’s been fed to them as input.
Quote unquote, training data, right? when machines are taught to do something, building models, they’re, they’re fed in training data. So there’s so much, uh, labeled training data where in we say, okay, this squiggle. is a six. That squiggle is a seven. And when you give thousands of those examples to these machines, you know, and to these algorithms, they actually then become really good at detecting what’s a six versus a seven.
Sometimes, as I mentioned in the translate example, even better than humans. Right. so that’s where, the first, huge [00:49:00] application of neural networks and all of that, you know, all of those techniques came about. Right. So what’s happened is, the ability to read these documents has improved, but then.
The ability to comprehend them too. So that exact that aspect of once you read all this, then saying, Oh, which of this is an asset, which of this is a liability, which of this, have there been defaults, have there been missed payments? These are the things that an underwriter or a loan officer is. You know, almost asking these questions or trying to get answers to these questions to feed into math model or whatever to come up with a decision of yay or nay, right?
So now, not only are you able to better read that document by virtue of machine learning, by virtue of all of these Models that recognize handwriting or forms. Now you’re also able to answer these questions. Uh, you’re able [00:50:00] to understand the data. so that’s, that’s the shift and it’s a real use case.
Uh, a few of, folks that I work with, a bunch of, Others in the industry, there’s tons of people who are helping out banks, insurance companies, a lot of companies actually automate, and take these, uh, workflows, which were heavily human, oriented, and, uh, almost acting like a copilot to them, right?
You’re never getting rid of the human, right? that’s the other myth that I just want to dispel. You’re making them. do what they’re doing in a lot more productive fashion in a lot faster, but ultimately you’re never getting rid of the human for the foreseeable future.
Josh: you know, talking about use cases that technology, you may, uh, or may not have better insight into this than I do, but I’ll tell you what, have you ever used the software Expensify?
Shekhar: Yeah. Yeah. Uh, for [00:51:00] 10 years, nine, 10 years. Yeah.
Josh: is one of
Shekhar: Receipts. Receipt scanning.
Josh: Yeah, I’ve made the joke. Yeah. So for those of you who don’t know, Expensify is, is an expense tool.
That’s everything from it actually, you know, can take the picture of your receipt or you can upload it in to creating the expense report, to pushing it to your accounting team. Review all processes like that whole nine yards. But what’s really special about it, and I’ve joked internally at Tyfone, I’m like having Expensify is a retention tool for me as an employee.
Like I would never leave just from the standpoint that I would never want to go to a company that didn’t use Expensify for my expense reports. because it does such a phenomenal job and like, I mean, I’ve been using it for a few years now and just consistently blown away at how well it can take such a crazy wide range of different form factors of receipts I put into it.
Everything from handwritten notes to, um, you know, [00:52:00] PDF folios from, You know, hotels to a receipt at a restaurant where you cross out the number cause you did the math wrong for the tip and then put a new one in and it takes all of that out, automatically fills out your expense report. And I mean, it is wildly rare how often it gets it wrong.
Although the best one I’ve ever had it get wrong once Shekhar, I thought it was pretty funny. I bought, I bought like an iPad for work. Um, for us to use it like a trade show or something, just add an Apple store and got the Apple receipt digitally, right? So it’s super nice, clean, digital, uploaded it into Expensify.
What’s an iPad? You know, I mean, a couple hundred bucks, right? But it auto filled my amount as like 6 trillion reimbursable. And I was like, Oh, absolutely. That is correct. Yes. Expensify. Please back me up. I deserve 6 trillion, uh, reimbursed, [00:53:00] please. Tyfone.
Shekhar: that’s the bug you were hoping for all your
Josh: yeah, that’s the bug I was hoping for this whole time.
Yeah. Um, but, but in all seriousness, I mean, it’s absolutely phenomenal at how good it is at reading all of the, my chicken scratch to, you know, more structured data. So I think that’s just, I use that as an example of. I think a lot of times too, we’re even interacting with some of this technology without even realizing it, but it’s, it’s finding the right use case and then not just saying like this one thing is going to solve all the problems for all the use cases.
And I know if you’ve heard me talk about this, you know, topic on the podcast before, I’m probably, overbeating this, this topic, but I think it’s so true, right? That we need. To understand that some of this new technology is absolutely a cheat code. Like this is a cheat code for the video game.
And if you’re not using it, you’re losing. Right. But at the same time you have to know how to apply it. [00:54:00] Right. And so saying like the car is a cheat code. Well, yeah, the car is a cheat code. If you have say a delivery business. Right. So it may revolutionize how your, you know, um, Pizza restaurant is able to deliver more product using the car faster.
But if you’re saying, like, I’m going to use the car to make more pizzas. It’s not a great tool for that, right? But it is a great tool for delivering the pizzas. And so if you’re, you know, pizza hut and you’re using horse and buggy to deliver pizzas and Domino’s is using cars like Domino’s just is going to sell more pizzas.
Right. But if all of a sudden Pizza Hut is like, well, we’re going to use cars like Domino’s, but we’re going to use them to make pizzas. Like, they ain’t going to work out so well. So it’s, it’s finding the right use case and applying it. But this is kind of the cheat code of our era, right?
Shekhar: it truly is. And there’s so many things that I just want to pass out of what you said, because there was, there’s a couple of things I was going to refer to too. So [00:55:00] first and foremost, I think, it’s a combination of two pieces, right? One is value. So, so make sure you’re understanding where the value is, right?
The opportunity, the potential, all of it, forget the technology, so for a bit, And I know places where they’ve said, Oh, I have 180 use cases. I can apply. I can apply this technology to, which is great, but where do you start? right. Uh, it’s, and no company with, even the biggest ones out there just don’t have enough talent to go after all this.
I mean, forget talent, even if they have talent, that talent does not have enough know how yet, yet being the key word, because we’re still, you know, early days, right? The best practices, the, the shared the mind share, the knowledge has just not kind of,trickled down or gone, gone across the board.
So, so first and foremost, you got to focus on where the value is. And second, to your [00:56:00] point, make sure that you’re, you know, not using this as that’s, the, everything looks like a nail cause I have a hammer. That cliche, but nobody knows that. very well, right where I see, the best of companies that succeed at this, uh, like, okay, I understand enough of this, but I acknowledge that I don’t know it all.
And hence, what we’ll do is we’ll now get into this, experimentation phase, the rapid iteration, not to actually say, Hey, we’ve solved a problem, but Right. You actually want to fail 10 times because that helps you, learn as to what’s good, what’s not good. That’s where, companies that are, that have that mindset, have that ability To, change and adapt are the ones that are really already churning out some value and realizing some of the value from tech like this, right?
I think this applies in [00:57:00] general to any innovation or tech, but specifically here, just because there’s such little understanding, and common knowledge. that’s the one benefit I see. I just want to go back to your example. that’s a classic, that’s a great place where, uh, it brings together those couple of, uh, foundational, bits that I was talking about.
What does, Expensify didn’t do this on day one, right? They did this over the last few years. But what preceded it? going back to your question, what was all this built upon they had for thousands and thousands of companies for, almost 5 to 6 plus years. They had millions of receipts that they, you know, people would upload the receipts as evidence.
So first ingredient check, which is tons of data, right? Second ingredient, [00:58:00] they actually did all the hard work for somebody, a bunch, a huge team to go through. And, you know, like classify, identify, categorize, painstakingly say, Oh, yeah, this is the store name in the receipt. This is the date of purchase amount.
All of that stuff. Somebody did all that hard work and then they just threw these algorithms and compute at it. Right? And, with enough confluence of all these things. That’s when you saw it kept on getting better every, you know, quarter. I still remember emails from, uh, the expensive CEO. he sends out these really nice emails, uh, talking about the product.
I don’t know if you ever, Yeah, I saw one. and, but, but that’s where innovation, techniques, plus all the hard work of bringing the data together and making it useful, that’s when, that’s when real value comes together. The last piece, actually, [00:59:00] another thing, going back to, you talked about, uh,you know, the, pizza hut, uh, using the, uh, you know, uh, car for, something that, uh, for making pizzas or somebody delivering using horse buggies. The other aspect, it’s a little bit of a, leap. But one of the key things, you know, everybody talks tech and after a while, tech is probably the easier part.
We’re all humans. So we bring together our strengths. We bring our strengths to the table, but we also have our weaknesses or biases and emotional, you side to this. of the biggest, concern everybody has is like, okay, what’s it gonna do to my job? Right? Uh, what am I, going to be out of work?
what’s going to happen? So, and. it’s of course not a pretty conversation because in the past when this used to come up, right, everybody’s been through the industrial age. you know, even, uh, so many of the manufacturing, uh, work going, offshore. [01:00:00] So, tons of transitions, but it’s not like.
We got out of work, but these, these things didn’t happen overnight. They happened over a period of, you know, , 10, 15, 20 years. Sometimes what’s scary. I think from all of this is just how quickly some of these things are going to happen. so going back, 2 aspects, right? 1 for anybody who’s listening.
I think the, especially if you’re early in your career, right? As much as it’s about picking skills up, it’s also knowing which skills will still be, valid and, you know, like still not be commoditized by all this tech versus which ones. Will be and making sure you’re also orienting yourselves to pick those up, right?
Because that’s a key aspect. Now, I don’t want to make this a lot more about, hey, what’s going to what’s the future of work? What’s going to happen? All of that. But but the other aspect is also I’ve seen a lot of. [01:01:00] from everybody saying, is this going to make us, you know, lazy or dumb or whatever, right?
I mean, how long ago was it that people had to remember addresses, phone, phone numbers, recipes, whatever it was. Who does that anymore? I mean, I still remember a few, but I not like, not more than three or four. Right.so. Why is that? But has it made us any worse off? No, we still adapt to, how things are.
So, so that’s the other aspect of we shouldn’t be concerned around the whole, uh, whole piece of, is this going to make us worse off and machines are going to take over? No, yes. In certain instances, we’ll, you know, we will probably become, fall behind a little bit. Okay. But human is always going to trump everything else.
That’s at least that’s how I see it. Or I’m a big believer of that.
Josh: I’m really glad you, you kind of brought up this part too. Because as you were talking about, just some of the different [01:02:00] examples of how, especially in banking and finance, you know, these tools are getting leveraged. It was interesting when you were talking about the whole stitch fix thing, one of the things that I was thinking about was, you know, let’s say stitch fix gets it wrong and they send me something that I would just never in a million years where.
Like, meh, no harm, no foul. Right? Especially if it’s only one out of a bunch and I’m like, ah, you know what? They got it wrong once, whatever. You know, they have a good return policy to send it back. No big deal. Great. But what happens if my bank or credit union, for example, starts to leverage this technology and says, Oh Josh, we think actually you should drain your entire checking and savings account and put it in this 12 month CD because you’d make more money than you are today. maths and then all of a sudden my mortgage comes due and I have no money to pay my mortgage and I’m not getting paid again until after my mortgage is due and now my mortgage is late or I have to take penalties on [01:03:00] withdrawing that CD, right? That was bad advice. That was harmful advice, right? And then as we start to look at, like you were using the example of, loan underwriting, right?
There’s absolutely human bias that is done today. But there’s also really great humans that are doing that today. And so how do we have an AI model that does a better job of removing biases and being truly objective about is this a loan that we should fund and underwrite? Yes or no. But at the same time, if whoever writes that program does a perfect job and it is absolutely flawless, except it hates everyone with the name Josh and just automatically denies them because they suck.
Now you’ve got another problem, right? As soon as we figure that out, us Joshes, man, we’re rising up. Class action lawsuit coming your way. so I think there’s also some. Both excitement and fear, especially in the [01:04:00] banking space around those tools. Just from those two standpoints as well. What do you think?
Shekhar: Yeah, absolutely. And this is so spot on, but at the same time, this isn’t new, right? So, I’ll take the example of, I won’t take names, but, uh, in a past life, worked with some, the top four bank in the U S all of it.
Till till even a few years ago, so obviously, first and foremost, two concerns, right? banks are heavily regulated. So, something that we don’t appreciate on a daily basis, but the amount of compliance and regulatory checks and, every time you’re, especially when you’re, you know, making decisions off of credit or loan or any of that kind of stuff where you could be denying somebody.
So just, tons of regulations around fair lending. Obviously it was concern, , when all this AI models, predictive models, machine learning models, came about [01:05:00] and they said, okay, you know, instead of your, uh, models that were built in a more traditional fashion by your, stats people, by your risk folks, everybody who ultimately then gave this very descriptive.
If this, then that kind of a thought process, to now a machine just learning what’s good and bad from past behavior, right? Which is awesome, but past what’s defined and encoded in the past behavior could have biases, right? so us as humans could be feeding it something that is not the best representation of reality.
Worse is not a fair representation, because so it, one’s one’s more about profit and loss. The other one’s more about regulatory challenges. So I think all of that still holds true. And that’s where. going back to the use case, one of [01:06:00] the key things is, any enterprise has to look at a multitude of dimensions and define those dimensions as being the, key, parameters on which it would evaluate the use case.
Right. So, so one of them I already mentioned, what’s the value? What’s the benefit? But then the flip side is what’s the risk? Is there risk from a customer experience perspective? I don’t know if you ever saw that, chatbot that Chevrolet had put up on their website, where, uh, it was, Yeah, these, these are the early days of, uh, you know, sometime, early to mid last year, everybody was coming up with ChatGPT back chat bots.
But then all it did was you could type in any prompt as an end user, right? So I could go to chevrolet.com, type in a prompt, and then, it would, you know, it would spit out whatever ChatGPT knew. Right, not Chevrolet’s point of view, but ChatGPT’s point of view. [01:07:00] So some, some folks took screenshots and it became a craze on Twitter too, or I guess X today, where somebody said, hey, tell me how Tesla is better than Chevrolet.
And this is on a chevrolet.com, right? I mean, uh, on a, on, their property and like screenshots after screenshots after screenshots flooding the internet. Now, okay, hiccups like this happen. You’ve heard. So that’s still a, that’s still a problem. Okay, not as harmful, but you’ve heard of the Microsoft Bot, which, you know, had a huge racial bias.
and when it was turned on, it started, you know, it just the quickest thing it learned was abusive language because the internet is so full of it. Uh,
Josh: we humans are kind of shitty sometimes and it picked up on that real quick.
Shekhar: Exactly, right? I mean, maybe somebody just fed it a bunch of YouTube comments because that’s like as bad as it gets, right? [01:08:00] But, but it just goes back to that aspect of what’s the risk? You really have to, put, an insane amount of thought into what’s the risk? What’s the exposure? What if something goes wrong?
Who is getting impacted? How, how do you address it? So that’s why. Everybody, I think, is still, you know, while they’re looking for extracting that value, but they’re also kind of playing it safe in some ways, by making sure that, you know, there’s a human in the loop, there’s somebody to review, there’s somebody to kind of, you know, To your point, be that gating function so that nothing bad happens.
While, while techniques are improving so that stuff like that doesn’t happen automatically. there’s lots of research around guardrails, all of it, but ultimately right now that is still a human in the loop is still king.
Josh: Well, I think that’s a good point too in that, probably especially in banking, right? there’s a wide spectrum of the risk versus [01:09:00] reward. And in some things we may be able to be, you know, the perceived risk of something going wrong is lower, right? I don’t know. Just trying to think of a, an example of, you know, asking somebody where the nearest branch is, and they send me to one that’s two miles away versus one that’s 1.
9 miles away. Like the risk is pretty low, right? But the risk of, you know, overriding all of our core data, for example, would probably be a very high risk. And so there are certain things where our risk tolerance is a complete and utter zero. There’s zero that we can accept. And so That’s probably gonna be a really long tail of having things like A.
- participate in those because the risk tolerance is zero, like we cannot have a screw up right at the same time. Well, with that scenario, if humans are involved, there’s always risk involved because we’re not perfect either. But you get what I’m saying. Um,
Shekhar: We always hold machines to higher standards than we hold ourselves.
Josh: [01:10:00] Well, in two, I think there’s, there’s the whole other conversation of like, if it screws up, who’s liable.
Right. and so, but at the same time, there’s probably things within financial institutions where we’re like, ah, you know what? The risk isn’t zero. Like we, we can accept a little bit of risk here. So to your point, like we can start to dabble, we can start to do things. you know, I, one other thing that you brought up, I don’t know if I’d ever really thought about until you were talking about.
and I’d love to get your take on is you were talking about one of the other challenges and why this, you know, this technology had not become quite so pervasive was that it was compute hungry, right? It takes up a lot of resources, and, you know, I think we’re seeing that in everything from things like Bitcoin mining to, you know, the energy it takes to run a supercomputer.
I think we’re also at an interesting, intersection of we’re trying to be, hopefully, a lot better about how we do things. take care of [01:11:00] this big, you know, rock that we all live on. That’s hurtling around in space. we kind of only get one, right? Unless Elon figures out the whole Mars thing. But I think, I think at least in our lifetime, like I’m stuck with this one.
So I kind of want to treat it pretty well. Right? So we’re trying to get better about treating this whole rock. We’re on a little bit better. And at the same time, we’re starting to do these things that are incredibly energy intensive. Is there, is a mix of. Desires in that from your perspective, Shekhar, like is this going to be something where we’re also going to run into just a limitations of we’re using more power than we really should to be doing some of these things.
I don’t know.
Shekhar: so I think there’s a short term answer and there’s a, you know, longer term answer. Right. So in the short term,
it’s, it’s definitely, uh, so, and this is where, uh, I’m seeing it date, you know, daily, increasingly, right. the ROR. What’s what’s unknown till [01:12:00] you do, I don’t know, a few dozen of these at a dozen places. It’s very difficult to articulate. Okay, I’m putting in all this money, to build this use case.
What’s the ROI? But ROI is two things. One is what, whatever, effort money went into building it, but also what’s going into running it, keeping it running. Right. So to your point, and that’s not cheap. I’ve seen some numbers and woof. Uh, it’s like, it’s, more capital investment, than, what we’ve, what technology teams have done for a long time, ever since you moved to the cloud.
CAPEX has reduced a lot, right? It’s gone into the OPEX mode. so, but the bills here can run up real quick if you don’t know what you’re doing, uh, if you aren’t careful. so, so that’s one aspect of, you know, your question, right? But, the other aspect, in the short term, it [01:13:00] is expensive. It is energy hungry, which means there is a, you know, there is, not all of the energy, powering.
All this is clean right now or green. so there is going to be a carbon footprint. I think we are also.uh, like it or not, we have kind of jumped onto the a, on the back of a Tiger and we are just gonna run with it. But where I am bullish and I see is a few things, two or three, which are, Relatively close to the ground and one which is out there. So, first and foremost, part of the reason why, and this is going to be slightly controversial, but, I, I do think and most people will probably over a period of time agree is first and foremost, the models themselves are still very inefficient, right?
Now by virtue of the way they’re built or by the virtue of the way they’re used or sometimes both but they are [01:14:00] right it takes this. Remember this it’s still very early days in terms of like Everybody is focused more on like how do I even build one to? Now the focus is shifting to how do I make sure that these things are actually used, right?
And used effectively. the price point is reducing all of that.so, so that’s 1st. The 2nd thing is, I mean, while there’s a reason why, NVIDIA, NVIDIA hit 3, 3, 3 trillion valuation, and it’s great for everybody’s stock portfolio, But two things, right? The competitions, you know, NVIDIA has always been good at staying ahead, but in general, there is going to be some competition that will help bring down the pricing too, right?
two things, because, it’s just Any place where there’s so much money and given capitalism, ultimately it does tend to happen. It may not, it may not bring down the price by [01:15:00] 10 times, but it will come down quite a bit. and this is more the, 18 to You know, 48 month timeline, right? For the next, uh, 9 to 18 months.
I think we’re still going to be in that space where slight reduction in cost every quarter, but not huge reduction in, and the other, the 3rd part, which is really out there, uh, which probably is still now. I know everybody said that that over the last 50 years. Nuclear fusion has been 30 years away. but I think that, you know, so to your point, yes, there’s, I don’t think there’s a ceiling, but the cost of energy, and the cost of a lot of these investments is going to throttle where all you apply this for a few years.
But by virtue of price points coming down and I’m a, I’m a huge believer in like, okay, we are again, probably two decades away from a [01:16:00] decade and a half to two decades away from something like nuclear fusion. and Once that happens, now, just imagine, right, the biggest roadblock that you had, it’s just unleashed.
So, so again, that’s for more of a, uh, much deeper, different conversation, but I do think that over a period of time. All of this is going to come down initially slowly, but eventually significantly.
Josh: I mean, I, I’m just the, you know, non techie over here, but I feel like have we not just asked ChatGPT, Hey, figure out a way to power yourself with green energy. Like, come on, is that not the solution here or what?
Shekhar: Well, unfortunately, it only can ultimately learn from what we’ve taught it, right? Otherwise, it’ll just see the thing is, it’ll give you a perfectly good answer. And this is important. I’m glad we came about to this in a different way. part of that reason why that, you know, that whole risk thing, all of it, right?
It’s, you know, Why, why are we not going [01:17:00] to apply over a period of time? I’ve heard from so many people. Oh, why don’t we use ChatGPT and Gen AI to do this? no, you cannot because, it’s still non deterministic, right? In the sense that, everything else about computers so far, you tell it X. And X plus Y is always going to be Z if that’s how you taught it.
But here you’ve, I’m sure folks have heard the term hallucination, but for anybody who has not, it’s the, you know, it’s the instance where You ask ChatGPT something, and in a bunch of instances, it will give you some, give you back an answer, which is based on truth, but it will happily, without batting an eyelid, assuming it was a person, it had a personality or, give you an entirely made up and incorrect answer.
Right. So, so that’s the other thing, risk from a risk perspective, or as you get as we as professionals [01:18:00] and, users get better, it’s also having an appreciation for where to apply this kind of stuff versus not because anywhere where even there’s that, uh, 0 percent tolerance to, hallucination or stuff like that.
You just cannot go there, right? Just imagine if you lied to or presented an incorrect, made up answer to one of your customers when they are on a critical call. it’s the risk is too high. So that’s why for the foreseeable future. We are still going to govern and, gate all the output for all of this tech.
Josh: I mean, this is fascinating stuff. I think now I want to run this episode and all my episodes, through an AI tool to tell me how much, um, I talk versus my guests because I’m pretty sure you’re going to take the cake. I think we’ve recorded now over an hour and a half and I think in or excluding your [01:19:00] intro, I think I’ve maybe talked four minutes this whole time.
This has been fascinating. That’s a good thing by the way. Um,
Shekhar: Well, I was worried.
I was worried if he would even go to 30 minutes.
Josh: Yeah, no, I’m like, it’s just, I think that’s what’s so cool. This is what I’ve loved about, the podcast is like getting people on who are so deeply passionate and, like educated on their subject matter that, I mean, you virtually needed nothing from me and just wrote your own whole episode on, our conversation today.
I mean, it’s just. Fascinating. but Shekhar before I end the podcast, I definitely have to ask this question of you because I’m genuinely very curious now, where do you go to get information? Like what, what kind of stuff do you pay attention to?
Shekhar: Oh, uh, so there’s different kinds and there’s no one source. But, so one thing I worked over the years is on my Google food. I can, I can out search the, [01:20:00] the other, that’s probably the one skill I have better than most folks. know, uh, which is probably what keeps me going, right? Makes allows me to fake it.
but that’s one thing because, especially. In a lot of instances, when you’re working with such a wide spectrum of stuff, it’s not easy to just always go to two or three authoritative sources, right? I mean, you won’t have that. So that’s one, but at the same time, there’s, especially for, uh, a lot of the technical stuff.
I, I do a lot of medium, blogs, follow a bunch, but, there’s a couple of websites, which I recommend anybody who’s in the tech space, especially, you know, uh, software programming, architecture, things like that. there’s infoq. com, there’s dzone. com, another place that, I love, and this is where I pick up trends and, What’s good.
What’s [01:21:00] upcoming. What’s actually materializing fairly well is there’s this company called ThoughtWorks. A few folks may have heard of it, but like another technology consulting company. they. every quarter they release a tech radar, right? So, anybody who’s interested in trends specifically around technology in the, like, you know, the software engineering and associated realm should go look up the tech radar from ThoughtWorks.
It’s amazing presentation, amazing articulation. So that’s another place that I
go to
Josh: that out.
Shekhar: Yeah, yeah. So highly recommend that.
Josh: Um, well, if people want to connect with you or if they want to learn a little bit more about EXL analytics, where and how can they do that?
Shekhar: Best place is LinkedIn. If you just type my name up there, I’ll You know, uh, it’s, unique enough to pop up at the top. just drop me a, a dm. I’m trying to get back [01:22:00] into Twitter a little bit, but, uh, LinkedIn is the best path, EXL. definitely hit up our website, EXLservice.com.
we do a lot of work at the intersection of, you know, we have huge domain expertise in banking, insurance, some of these, doing this for 15, 20, 25 years, at the intersection of domain, data and, uh, AI, right? So, helping our customers, essentially bring all this together and realize value.
So, uh, look us up on, uh, EXLservice.Com.
Josh: Awesome. Hey, Shekhar. Thank you so much for coming and just sharing so much knowledge with us. I feel like, I’ve had lots of conversations about AI over the last handful of, years. It’s really picked up in the last little bit. I am absolutely walking away with, I think, a much better understanding. So I really appreciate you taking the time and coming and being a guest on the digital banking podcast.
Shekhar: Thank you, Josh, for [01:23:00] being so kind with your words. Uh, I have to say this was especially enjoyable. thank you for making this, you know, so easy. And, really, as we discussed just a conversation, thank you. Uh, good luck to your future, for your future episodes too.
Thanks again.
Josh: got a high bar to hold after this one. So
Shekhar: Oh, you’re, you’re too kind,
Josh: no, seriously. I’m like, man, this is going to be one of those ones that my brain’s going to hurt afterwards. We should have scheduled this later in the day for me so I could be done after this. No, seriously. Thank you again. This was a really, really phenomenally educational episode for me.
Shekhar: Thank you. I’m glad. hopefully, uh, some of the audience finds it like that, too. So thanks.
Josh: I’m sure they were.
[01:24:00]